How Executive Leaders Can Leverage the Data Rush
More than three decades after the term big data entered the vernacular, cloud-technology and smart devices have propelled the world into a new phase of analytics. The growing network of internet enabled devices has turned every person into an individual data generator. The abundance and ease of access to big data has presented a novel challenge to companies to conduct effective data-driven decision making. Leaders who can identify and apply data-driven approaches to their strategic growth and business operations are well prepared for the endless possibilities of the big data era.
Consider Innovative Data Solutions
While interest in harnessing big data is prevalent across industries, data collection alone is not sufficient for analysis or data-driven decision making. In fact, raw data can actually drive down profits by projecting inaccurate forecasts, or simply not yielding any useful information.
Businesses need data processing and analytic software to capture and consolidate and transform big data into searchable data sets and visual presentations. However, as effective data analysis requires tailored solutions, tools for niche markets have become a booming industry. For example, nonprofit and church dashboards present vital metrics such as donation reports and impact indicators.
First movers in the data solution space have the ability to tap into the burgeoning global big data and business analytics industry, which is set to skyrocket from a market value of just under $200 billion to more than $650 billion within the next five years.
Although many of the biggest players in the analytics software sector are cloud-native companies, any enterprise can leverage its expertise and knowledge to create an industry-specific data solution.
Companies like Wix and Pegasystems incubate innovative data processing solutions by testing software in-house that are later sold as B2B products. This cycle of building, testing, and then selling in-house tools is an effective avenue for companies in any sector to prototype solutions and test market viability.
Include Data from the Top Down
Data-driven corporate cultures don’t just happen. Instead, innovation is advanced by open-minded and informed leadership. In the early days of business analytics, companies largely delegated data collection and decision-making to a sole technology savvy executive or department. Today, most leaders recognize that an integrated data-first approach that activates and involves all stakeholders is necessary for cultivating the best results.
Shifting to a data-driven culture often means overhauling multiple aspects of a company’s strategy. Executives who usually rely on their intuition must fully embrace data’s role in validating, expanding, or negating business decisions.
At the same time, data analysis is meaningless without context, experience, and insight. The key is to view data as a launch pad for deeper investigations. This mindset avoids common pitfalls such as cherry picking or blind reliance on numbers.
Education is a cornerstone in successful data-driven companies. Top firms such as Eli Lilly and TD Bank have incorporated data analysis training into their executive professional development programs.
Trained leaders can support and encourage an ecosystem of continuous learning within their departments and teams, thus fostering an environment that is conducive to the dynamic nature of a data-driven business.
Focus on Quality over Quantity
Considering the unimaginable volumes of data generated each day, (as much as 2.5 quintillion bytes), the term big data is a bit underwhelming. While the ability to capture unstructured data has made innovative insights possible, it has also highlighted the importance of data quality. Data driven leaders must acknowledge the limits of data in order to leverage data-driven results to their full potential.
How data quality is defined and assessed is highly nuanced. A basic principle of data analysis asserts that high data volumes reduce the effect of anomalies and errors. When tracking historical trends or longitudinal outcomes, prioritizing quantity can make sense.
However, as most companies are using data to make business decisions that impact individuals or a more refined demographic, data quality often surpasses quantity. This is particularly salient if a company wants to have a better understanding of a specific customer segment, market, or product performance. Employing data sampling methods can cut out some of the background noise and uncover insights that would otherwise get lost.
Analysts agree that data quality is only as superior as its source. And since humans select inputs and metrics, any data analysis has the potential to reflect flawed biases. Artificial intelligence and machine learning tools are notorious for replicating many of the same distortions companies have turned to algorithms to erase, including prejudices and cognitive biases. Relatedly, removing the human touch from the analysis process altogether runs the risk of alienating customers and disincentivizing employee innovation. Following the axiom “people first, data second” can help leaders navigate the often blurred lines between helpful and harmful analytic processes.
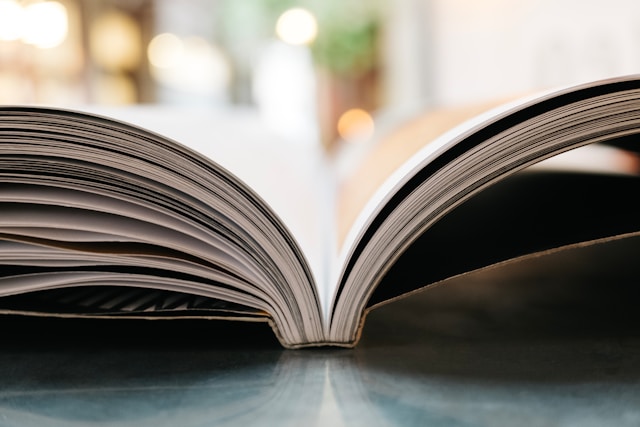
Stay updated with our latest publications.
Discover Issues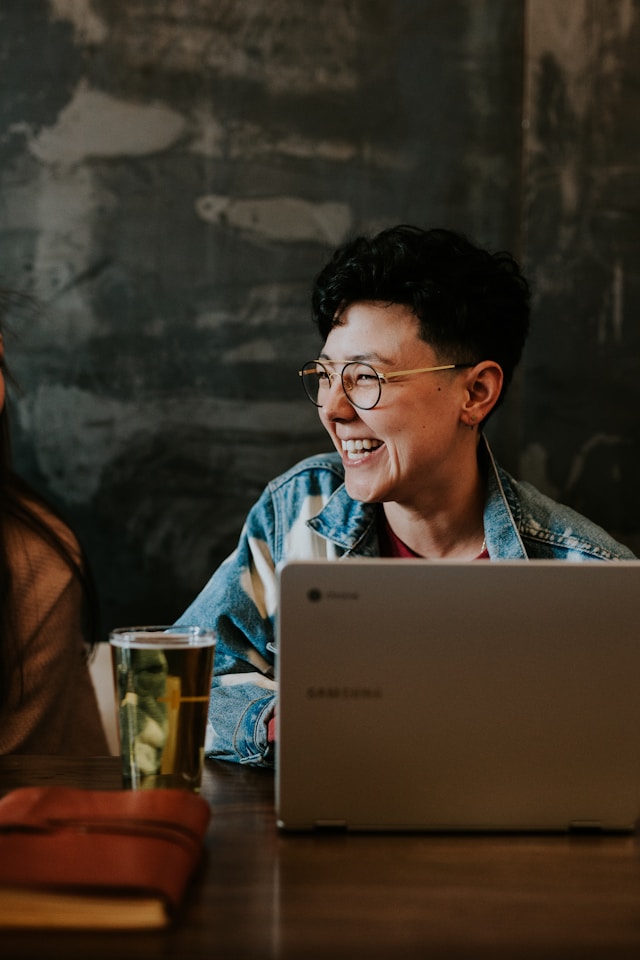
See how we can help you grow in the online space!
Advertise With Us
We can help promote your business.
Find Out More